Llei dels grans nombres: diferència entre les revisions
m robot estandarditzant mida de les imatges, localitzant i simplificant codi |
Ampliació via traducció (inacabada) de la versió anglesa. Edició en procés. |
||
Línia 1: | Línia 1: | ||
{{FR|data=abril de 2019}} |
{{FR|data=abril de 2019}} |
||
{{traducció|en|Law of large numbers|18/11/2019}} |
|||
[[Fitxer:Largenumbers.svg|miniatura|Una il·lustració de la llei dels grans nombres, amb una sèrie concreta de llançaments d'un [[dau]]. Conforme augmenta el nombre de llançaments, la mitjana dels valors de tots el resultats s'aproxima a 3,5. Mentre que sèries diferents de llançaments poden mostrar un esquema diferent quan ecara s'han fet pocs llançaments (a l'esquerra), quan augmenta el nombre de llançaments (a la dreta) les sèries es comporten de manera similar.]] |
[[Fitxer:Largenumbers.svg|miniatura|Una il·lustració de la llei dels grans nombres, amb una sèrie concreta de llançaments d'un [[dau]]. Conforme augmenta el nombre de llançaments, la mitjana dels valors de tots el resultats s'aproxima a 3,5. Mentre que sèries diferents de llançaments poden mostrar un esquema diferent quan ecara s'han fet pocs llançaments (a l'esquerra), quan augmenta el nombre de llançaments (a la dreta) les sèries es comporten de manera similar.]] |
||
En [[teoria de la probabilitat]], la '''llei dels grans nombres''' és un teorema segons el qual quan el nombre d'observacions d'un fenomen aleatori és molt gran, la freqüència d'un esdeveniment associat amb aquest s'aproxima progressivament a un valor determinat. Aquest valor s'anomena [[probabilitat]] de l'esdeveniment. |
En [[teoria de la probabilitat]], la '''llei dels grans nombres''' és un teorema segons el qual quan el nombre d'observacions d'un fenomen aleatori és molt gran, la freqüència d'un esdeveniment associat amb aquest s'aproxima progressivament a un valor determinat. Aquest valor s'anomena [[probabilitat]] de l'esdeveniment. |
||
Línia 6: | Línia 7: | ||
* Comprovar si són vàlides o no les probabilitats assignades a priori als esdeveniments dels instruments aleatoris suposadament regulars. |
* Comprovar si són vàlides o no les probabilitats assignades a priori als esdeveniments dels instruments aleatoris suposadament regulars. |
||
* Obtenir de manera aproximada les probabilitats d'esdeveniments d'experiències aleatòries irregulars. |
* Obtenir de manera aproximada les probabilitats d'esdeveniments d'experiències aleatòries irregulars. |
||
Aquesta llei és important perquè garanteix relacions estables entre les [[Mitjana (matemàtiques)|mitjanes]] de diversos esdeveniments aleatoris. Per exemple, mentre que un casino pot perdre diners en una simple tirada de la [[ruleta]], els seus guanys tendiran a un percentatge predictible amb un nombre gran de tirades. Qualsevol tongada sort del jugador serà, eventualment, superada pels paràmetres del joc. Cal recordar que la llei, però, tan sols s'aplica quan es considera un nombre elevat d'observacions, tal com el nom indica. El principi no es pot aplicar per un nombre petit d'observacions ni es pot esperar que una tongada d'un valor concret sigui immediatament "equilibrada" amb l'obtenció d'altres valors (consulta la [[fal·làcia del jugador]]). |
|||
==Exemples== |
|||
Per exemple, una única tirada d'un dau equilibrat de sis cares té sis possibles resultats (1, 2, 3, 4, 5 o 6), tots amb la mateixa probabilitat. Per tant, el valor esperat de la mitjana de tirades és de: |
|||
<math> \frac{1+2+3+4+5+6}{6} = 3.5</math> |
|||
Segons la llei dels grans nombres, si es tiren un nombre elevat de daus de 6 cares, la mitjana dels seus valors serà pròxima a 3.5 i la seva precisió augmentarà amb la tirada de més daus. |
|||
D'aquesta llei, se'n pot deduir que la [[probabilitat empírica]] d'un [[succés]] o esdeveniment en una sèrie d'[[Assaig de Bernoulli|assajos de Bernoulli]] convergiran a la probabilitat teòrica. Per una [[Distribució de Bernoulli|variable aleatòria de Bernoulli]], el valor esperat després d'un nombre d'assajos prou elevat coincideix amb la probabilitat teòrica de l'esdeveniment i la mitjana de les ''n'' variables (assumint que són [[Variables aleatòries independents i idènticament distribuïdes|aleatòries, independents i idènticament distribuïdes]] (i.i.d.)) çes precisament la freqüència relativa. |
|||
Per exemple, la tirada d'una moneda equilibrada (on la probabilitat d'obtenir cada cara és igual), és un assaig de Bernoulli. Quan es tira per primer cop, la probabilitat de cara és 1/2. Per tant, segons la llei dels grans nombres, la proporció de cares en un nombre "gran" de tirades "hauria de ser" aproximadament 1/2. En concret, la proporció de cares després de ''n'' tirades convergirà de forma ''quasisegura'' cap a 1/2 a mesura que ''n'' s'apropa a infinit. |
|||
Malgrat que la proporció de cara (i creu) s'apropa a 1/2, a mesura que creix el nombre de tirades, pràcticament segur que la diferència absoluta entre el nombre de cares i creus també creixerà. D'altra banda, també pràcticament segur que el ràtio de la diferència absoluta respecte el nombre de tirades s'aproximarà a zero. De forma inuïtiva, la diferència absoluta esperada creix, però a un ritme menor que el nombre de tirades.<br /> |
|||
==History== |
|||
[[File:DiffusionMicroMacro.gif|thumb|right|250px|[[Molecular diffusion|Diffusion]] is an example of the law of large numbers. Initially, there are [[solution|solute]] molecules on the left side of a barrier (magenta line) and none on the right. The barrier is removed, and the solute diffuses to fill the whole container.<br> |
|||
<u>Top:</u> With a single molecule, the motion appears to be quite random.<br> |
|||
<u>Middle:</u> With more molecules, there is clearly a trend where the solute fills the container more and more uniformly, but there are also random fluctuations.<br> |
|||
<u>Bottom:</u> With an enormous number of solute molecules (too many to see), the randomness is essentially gone: The solute appears to move smoothly and systematically from high-concentration areas to low-concentration areas. In realistic situations, chemists can describe diffusion as a deterministic macroscopic phenomenon (see [[Fick's law]]s), despite its underlying random nature.]] |
|||
El matemàtic italià [[Gerolamo Cardano]] (1501–1576) va constatar, sense demostrar, que la precisió de les mesures estadístiques empíriques tendien a millorar amb el nombre d'assajos<ref>Mlodinow, L. ''The Drunkard's Walk.'' New York: Random House, 2008. p. 50.</ref> i es va formalitzar com a llei dels grans nombres. Una forma especial d'aquesta llei, per les variables aleatòries binàries) va ser demostrar primerament per [[Jacob Bernoulli]].<ref>Jakob Bernoulli, ''Ars Conjectandi: Usum & Applicationem Praecedentis Doctrinae in Civilibus, Moralibus & Oeconomicis'', 1713, Chapter 4, (Translated into English by Oscar Sheynin)</ref> Li va costar més de 20 anys desenvolupar una prova matemàtica suficientment rigorosa, que va ser publicada en la seva obra ''Ars Conjectandi'' (L'art de la conjentura) el 1713. El va anomenar el seu "Teorema daurat", però va ser conegut generalment amb el nom del "Teorema de Bernoulli", que no s'ha de confondre amb el [[Principi de Bernoulli]], referit al seu cosí [[Daniel Bernoulli]]. El 1837, [[Siméon Denis Poisson|S.D. Poisson]] el va desenvolupar més sota el nom "''la loi des grands nombres''" ("La llei dels grans nombres").<ref>Poisson names the "law of large numbers" (''la loi des grands nombres'') in: S.D. Poisson, ''Probabilité des jugements en matière criminelle et en matière civile, précédées des règles générales du calcul des probabilitiés'' (Paris, France: Bachelier, 1837), [https://books.google.com/books?id=uovoFE3gt2EC&pg=PA7#v=onepage p. 7]. He attempts a two-part proof of the law on pp. 139–143 and pp. 277 ff.</ref><ref>Hacking, Ian. (1983) "19th-century Cracks in the Concept of Determinism", ''Journal of the History of Ideas'', 44 (3), 455-475 {{jstor|2709176}}</ref> D'aleshores encà, es coneix pels dos noms, tot i que la "Llei dels grans nombres" és més freqüent. |
|||
Després que Benroulli i Poisson publiquessin els seus esforços, altres matemàtics van contribuir a refinar la llei, com [[Pafnuty Chebyshev|Chebyshev]],<ref>{{Cite journal | last1 = Tchebichef | first1 = P. | title = Démonstration élémentaire d'une proposition générale de la théorie des probabilités | doi = 10.1515/crll.1846.33.259 | journal = Journal für die reine und angewandte Mathematik | volume = 1846 | issue = 33 | pages = 259–267 | year = 1846 | pmid = | pmc = | url = https://zenodo.org/record/1448850/files/article.pdf }}</ref> [[Andrey Markov|Markov]], [[Émile Borel|Borel]], [[Francesco Paolo Cantelli|Cantelli]] and [[Andrey Kolmogorov|Kolmogorov]] i [[Aleksandr Khinchin|Khinchin]]. Markov va demostrar que la llei es podia aplicar a variables aleatòries que no tinguessin una [[variància]] finita sota alguna altra hipòtesi més feble, i Khinchin va provar, el 1929, que si la sèrie consistia en variables aleatòries independents i idènticament distribuides, era suficient que el valor esperat existis per tal que la llei feble dels grans nombres fos veritat.<ref name=EncMath>{{cite web|author1=Yuri Prohorov|authorlink1=Yuri Vasilyevich Prokhorov|title=Law of large numbers|url=https://www.encyclopediaofmath.org/index.php/Law_of_large_numbers| website=Encyclopedia of Mathematics}}</ref> Com a conseqüència d'aquests nous estudis, van sorgir dos formes prominents de la Llei dels grans nombres. Una anomenada la llei "feble" i l'altra la llei "forta", en referència a dos formes diferents de la convergència de la mitjana cumulativa de mostres cap al valor infinit. Tal com s'explica més endavant, la forma forta implica la feble.{{sfn|Seneta|2013}} |
|||
==Formes== |
|||
Existeixen dues versions diferents de la '''llei dels grans nombres''': la '''llei forta''' dels grans nombres i la '''llei feble''' dels grans nombres. Per una seqüència infinita de variables aleatòries [[Integral de Lebesgue|'''Lebesgue integrables''']] ''X''<sub>1</sub>, ''X''<sub>2</sub>, ... amb valor esperat E(''X''<sub>1</sub>) = E(''X''<sub>2</sub>) = ...= ''µ'', ambdues versions de la llei afirmen que, amb certesa virtual, la mitjana mostral, definida com a |
|||
:<math>\overline{X}_n=\frac1n(X_1+\cdots+X_n) </math> |
|||
convergeix al valor esperat |
|||
{{NumBlk|:|<math>\begin{matrix}{}\\ |
|||
\overline{X}_n \, \to \, \mu \qquad\textrm{for}\qquad n \to \infty, |
|||
\\{}\end{matrix}</math>|{{EquationRef|llei 1}}}} |
|||
La integrabilitat de Lebesgue per ''X<sub>j</sub>'' significa que el valor esperat E(''X<sub>j</sub>'') existeix seguint la [[Integral de Lebesgue]] i és finit. No implica que la mesura de probabilitat associada sigui continua absolutament respecte la mesura de Lebesgue. |
|||
La hipòtesi de [[variància]] finita Var(''X''<sub>1</sub>) = Var(''X''<sub>2</sub>) = ... = ''σ''<sup>2</sup> < ∞ '''no és necessària'''. Una variància gran o infinita farà que la convergència sigui més lenta, però la llei dels grans nombres es manté de tota manera. Aquesta hipòtesi, però, sovint s'usa per tal de fer la demostració més fàcil i més curta. |
|||
La [[Independència estadística|independència]] mútua de les variables aleatòries pot ser substituïda per la independència parell a en ambdues versoins de la llei.<ref>{{cite journal|last1=Etemadi|first1=N.Z.|title=An elementary proof of the strong law of large numbers|journal=Wahrscheinlichkeitstheorie Verw Gebiete|date=1981|volume=55|issue=1|pages=119–122|doi=10.1007/BF01013465}}</ref> |
|||
La diferència entre la versió de la llei feble i la forta rau en la manera en què la convergència és definida. |
|||
===Llei feble=== |
|||
[[File:Lawoflargenumbersanimation2.gif|thumb|Simulation illustrating the law of large numbers. Each frame, a coin that is red on one side and blue on the other is flipped, and a dot is added in the corresponding column. A pie chart shows the proportion of red and blue so far. Notice that while the proportion varies significantly at first, it approaches 50% as the number of trials increases.]] |
|||
The '''weak law of large numbers''' (also called [[Aleksandr Khinchin|Khinchin]]'s law) states that the sample average [[Convergence in probability|converges in probability]] towards the expected value<ref>{{harvnb|Loève|1977|loc=Chapter 1.4, p. 14}}</ref> |
|||
{{NumBlk|:|<math>\begin{matrix}{}\\ |
|||
\overline{X}_n\ \xrightarrow{P}\ \mu \qquad\textrm{when}\ n \to \infty. |
|||
\\{}\end{matrix}</math>|{{EquationRef|law. 2}}}} |
|||
That is, for any positive number ''ε'', |
|||
: <math> |
|||
\lim_{n\to\infty}\Pr\!\left(\,|\overline{X}_n-\mu| > \varepsilon\,\right) = 0. |
|||
</math> |
|||
Interpreting this result, the weak law states that for any nonzero margin specified, no matter how small, with a sufficiently large sample there will be a very high probability that the average of the observations will be close to the expected value; that is, within the margin. |
|||
As mentioned earlier, the weak law applies in the case of i.i.d. random variables, but it also applies in some other cases. For example, the variance may be different for each random variable in the series, keeping the expected value constant. If the variances are bounded, then the law applies, as shown by [[Pafnuty Chebyshev|Chebyshev]] as early as 1867. (If the expected values change during the series, then we can simply apply the law to the average deviation from the respective expected values. The law then states that this converges in probability to zero.) In fact, Chebyshev's proof works so long as the variance of the average of the first ''n'' values goes to zero as ''n'' goes to infinity.<ref name=EncMath/> As an example, assume that each random variable in the series follows a [[Gaussian distribution]] with mean zero, but with variance equal to <math>2n/\log(n+1)</math>, which is not bounded. At each stage, the average will be normally distributed (as the average of a set of normally distributed variables). The variance of the sum is equal to the sum of the variances, which is [[asymptotic]] to <math>n^2/\log n</math>. The variance of the average is therefore asymptotic to <math>1/\log n</math> and goes to zero. |
|||
An example where the law of large numbers does ''not'' apply is the [[Cauchy distribution]]. Another example is where the random numbers equal the tangent of an angle uniformly distributed between −90° and +90°. The [[median]] is zero, but the expected value does not exist, and indeed the average of ''n'' such variables has the same distribution as one such variable. It does not converge in probability towards zero (or any other value) as ''n'' goes to infinity. |
|||
There are also examples of the weak law applying even though the expected value does not exist. See [[#Differences between the weak law and the strong law]]. |
|||
===Strong law=== |
|||
The '''strong law of large numbers''' states that the sample average [[Almost sure convergence|converges almost surely]] to the expected value<ref>{{harvnb|Loève|1977|loc=Chapter 17.3, p. 251}}</ref> |
|||
{{NumBlk|:|<math>\begin{matrix}{}\\ |
|||
\bar{X}_n\ \xrightarrow{\text{a.s.}}\ \mu \qquad\textrm{when}\ n \to \infty. |
|||
\\{}\end{matrix}</math>|{{EquationRef|law. 3}}}} |
|||
That is, |
|||
: <math> |
|||
\Pr\!\left( \lim_{n\to\infty}\bar{X}_n = \mu \right) = 1. |
|||
</math> |
|||
What this means is that the probability that, as the number of trials ''n'' goes to infinity, the average of the observations converges to the expected value, is equal to one. |
|||
The proof is more complex than that of the weak law.<ref>{{cite web|url=http://terrytao.wordpress.com/2008/06/18/the-strong-law-of-large-numbers/ |title=The strong law of large numbers – What's new |publisher=Terrytao.wordpress.com |date= |accessdate=2012-06-09}}</ref> This law justifies the intuitive interpretation of the expected value (for Lebesgue integration only) of a random variable when sampled repeatedly as the "long-term average". |
|||
Almost sure convergence is also called strong convergence of random variables. This version is called the strong law because random variables which converge strongly (almost surely) are guaranteed to converge weakly (in probability). The strong law implies the weak law but not vice versa, when the strong law conditions hold the variable converges both strongly (almost surely) and weakly (in probability). |
|||
However the weak law is known to hold in certain conditions where the strong law does not hold and then the convergence is only weak (in probability).{{clarify|reason=Warning: This is ambiguous. By using the fuzzy word "may", it says that EITHER the weak law is known to hold under broader conditions than the strong law, OR the weak law is known to hold under certain conditions where the strong law is not known to hold, OR it is not known whether the weak law holds under broader conditions that the strong law. |date=November 2016}} |
|||
<!--The reference provided for the following doesn't seem to say this: |
|||
There are different views among mathematicians whether the two laws could be unified to one law, thereby replacing the weak law.<ref name="Testing Statistical Hypotheses">{{cite book|url=https://books.google.com/books?id=-kzPBAAAQBAJ&pg=PA219&lpg=PA219#v=onepage&q=is%20of%20very%20limited%20interest%20and%20should%20be%20replaced%20by%20the%20more%20precise%20and%20more%20useful%20strong%20law%20of%20large%20numbers | title=Law of large numbers views }}</ref> |
|||
To date it has not been possible to prove that the strong law conditions are the same as those of the weak law.{{citation needed|date=March 2016}} |
|||
--> |
|||
The strong law of large numbers can itself be seen as a special case of the [[Ergodic theory#Ergodic theorems|pointwise ergodic theorem]]. |
|||
The strong law applies to independent identically distributed random variables having an expected value (like the weak law). This was proved by Kolmogorov in 1930. It can also apply in other cases. Kolmogorov also showed, in 1933, that if the variables are independent and identically distributed, then for the average to converge almost surely on ''something'' (this can be considered another statement of the strong law), it is necessary that they have an expected value (and then of course the average will converge almost surely on that).<ref name=EMStrong>{{cite web|author1=Yuri Prokhorov|title=Strong law of large numbers|url=https://www.encyclopediaofmath.org/index.php/Strong_law_of_large_numbers|website=Encyclopedia of Mathematics}}</ref> |
|||
If the summands are independent but not identically distributed, then |
|||
: <math> |
|||
\bar{X}_n - \operatorname{E}\big[\bar{X}_n\big]\ \xrightarrow{\text{a.s.}}\ 0, |
|||
</math> |
|||
provided that each ''X''<sub>''k''</sub> has a finite second moment and |
|||
: <math> |
|||
\sum_{k=1}^{\infty} \frac{1}{k^2} \operatorname{Var}[X_k] < \infty. |
|||
</math> |
|||
This statement is known as ''Kolmogorov's strong law'', see e.g. {{harvtxt|Sen|Singer|1993|loc=Theorem 2.3.10}}. |
|||
An example of a series where the weak law applies but not the strong law is when ''X<sub>k</sub>'' is plus or minus <math>\sqrt{k/\log\log\log k}</math> (starting at sufficiently large ''k'' so that the denominator is positive) with probability 1/2 for each.<ref name=EMStrong/> The variance of ''X<sub>k</sub>'' is then <math>k/\log\log\log k.</math> Kolmogorov's strong law does not apply because the partial sum in his criterion up to ''k=n'' is asymptotic to <math>\log n/\log\log\log n</math> and this is unbounded. |
|||
If we replace the random variables with Gaussian variables having the same variances, namely <math>\sqrt{k/\log\log\log k},</math> then the average at any point will also be normally distributed. The width of the distribution of the average will tend toward zero (standard deviation asymptotic to <math>1/\sqrt{2\log\log\log n}</math>), but for a given ε, there is probability which does not go to zero with ''n'' that the average sometime after the ''n''th trial will come back up to ε. Since this probability does not go to zero {{clarify|reason=How can we see this does not go to zero? |date=October 2018}}, it must have a positive lower bound ''p''(ε), which means there is a probability of at least ''p''(ε) that the average will attain ε after ''n'' trials. It will happen with probability ''p''(ε)/2 before some ''m'' which depends on ''n''. But even after ''m'', there is still a probability of at least ''p''(ε) that it will happen. (This seems to indicate that ''p''(ε)=1 and the average will attain ε an infinite number of times.) |
|||
===Differences between the weak law and the strong law=== |
|||
The ''weak law'' states that for a specified large ''n'', the average <math style="vertical-align:-.35em">\overline{X}_n</math> is likely to be near ''μ''. Thus, it leaves open the possibility that <math style="vertical-align:-.4em">|\overline{X}_n -\mu| > \varepsilon</math> happens an infinite number of times, although at infrequent intervals. (Not necessarily <math style="vertical-align:-.4em">|\overline{X}_n -\mu| \neq 0</math> for all n). |
|||
The ''strong law'' shows that this [[almost surely]] will not occur. In particular, it implies that with probability 1, we have that for any {{nowrap|''ε'' > 0}} the inequality <math style="vertical-align:-.4em">|\overline{X}_n -\mu| < \varepsilon</math> holds for all large enough ''n''.<ref>{{harvtxt|Ross|2009}}</ref> |
|||
The strong law does not hold in the following cases, but the weak law does.<ref name="Weak law converges to constant">{{cite book|url=https://books.google.com/?id=K6t5qn-SEp8C&pg=PA432&lpg=PA432&q=%22even%20if%20the%20mean%20does%20not%20exist%22 | title=Weak law converges to constant | isbn=9780387276052 | last1=Lehmann | first1=Erich L | last2=Romano | first2=Joseph P | date=2006-03-30 }}</ref><ref>{{cite web|title=A NOTE ON THE WEAK LAW OF LARGE NUMBERS FOR EXCHANGEABLE RANDOM VARIABLES|url=http://www.mathnet.or.kr/mathnet/kms_tex/31810.pdf|publisher=Dguvl Hun Hong and Sung Ho Lee}}</ref><ref>{{cite web|title=weak law of large numbers: proof using characteristic functions vs proof using truncation VARIABLES|url=https://math.stackexchange.com/q/266870 }}</ref> |
|||
1. Let X be an [[Exponential distribution|exponentially]] distributed random variable with parameter 1. The random variable <math>\sin(X)e^X X^{-1}</math> has no expected value according to Lebesgue integration, but using conditional convergence and interpreting the integral as a [[Dirichlet integral]], which is an improper [[Riemann integral]], we can say: |
|||
:<math> E\left(\frac{\sin(X)e^X}{X}\right) =\ \int_{0}^{\infty}\frac{\sin(x)e^x}{x}e^{-x}dx = \frac{\pi}{2} </math> |
|||
2. Let x be [[Geometric distribution|geometric]] distribution with probability 0.5. The random variable <math>2^X(-1)^X X^{-1}</math> does not have an expected value in the conventional sense because the infinite [[Series (mathematics)|series]] is not absolutely convergent, but using conditional convergence, we can say: |
|||
:<math> E\left(\frac{2^X(-1)^X}{X}\right) =\ \sum_{1}^{\infty}\frac{2^x(-1)^x}{x}2^{-x}=-\ln(2) </math> |
|||
3. If the cumulative distribution function of a random variable is |
|||
:<math> 1-F(x)=\frac{e}{2x\ln(x)},x \ge e </math> |
|||
:<math> F(x)=\frac{e}{-2x\ln(-x)},x \le -e </math> |
|||
:then it has no expected value, but the weak law is true.<ref>{{cite web|last1=Mukherjee|first1=Sayan|title=Law of large numbers|url=http://www.isds.duke.edu/courses/Fall09/sta205/lec/lln.pdf|access-date=2014-06-28|archive-url=https://web.archive.org/web/20130309032810/http://www.isds.duke.edu/courses/Fall09/sta205/lec/lln.pdf|archive-date=2013-03-09|url-status=dead}}</ref><ref>{{cite web|last1=J. Geyer|first1=Charles|title=Law of large numbers|url=http://www.stat.umn.edu/geyer/8112/notes/weaklaw.pdf}}</ref> |
|||
===Uniform law of large numbers=== |
|||
Suppose ''f''(''x'',''θ'') is some [[Function (mathematics)|function]] defined for ''θ'' ∈ Θ, and continuous in ''θ''. Then for any fixed ''θ'', the sequence {''f''(''X''<sub>1</sub>,''θ''), ''f''(''X''<sub>2</sub>,''θ''), ...} will be a sequence of independent and identically distributed random variables, such that the sample mean of this sequence converges in probability to E[''f''(''X'',''θ'')]. This is the ''pointwise'' (in ''θ'') convergence. |
|||
The '''uniform law of large numbers''' states the conditions under which the convergence happens ''uniformly'' in ''θ''. If<ref>{{harvnb|Newey|McFadden|1994|loc=Lemma 2.4}}</ref><ref>{{cite journal|doi=10.1214/aoms/1177697731|title=Asymptotic Properties of Non-Linear Least Squares Estimators|year=1969|last1=Jennrich|first1=Robert I.|journal=The Annals of Mathematical Statistics|volume=40|issue=2|pages=633–643}}</ref> |
|||
# Θ is compact, |
|||
# ''f''(''x'',''θ'') is continuous at each ''θ'' ∈ Θ for [[Almost everywhere|almost all]] ''x''s, and measurable function of ''x'' at each ''θ''. |
|||
# there exists a [[Dominated convergence theorem|dominating]] function ''d''(''x'') such that E[''d''(''X'')] < ∞, and |
|||
#: <math> \left\| f(x,\theta) \right\| \leq d(x) \quad\text{for all}\ \theta\in\Theta.</math> |
|||
Then E[''f''(''X'',''θ'')] is continuous in ''θ'', and |
|||
: <math> |
|||
\sup_{\theta\in\Theta} \left\| \frac1n\sum_{i=1}^n f(X_i,\theta) - \operatorname{E}[f(X,\theta)] \right\| \xrightarrow{\mathrm{a.s.}} \ 0. |
|||
</math> |
|||
This result is useful to derive consistency of a large class of estimators (see [[Extremum estimator]]). |
|||
===Borel's law of large numbers=== |
|||
'''Borel's law of large numbers''', named after [[Émile Borel]], states that if an experiment is repeated a large number of times, independently under identical conditions, then the proportion of times that any specified event occurs approximately equals the probability of the event's occurrence on any particular trial; the larger the number of repetitions, the better the approximation tends to be. More precisely, if ''E'' denotes the event in question, ''p'' its probability of occurrence, and ''N<sub>n</sub>''(''E'') the number of times ''E'' occurs in the first ''n'' trials, then with probability one,<ref>[https://www.jstor.org/discover/10.2307/2323947?uid=3738032&uid=2&uid=4&sid=21103621939777 An Analytic Technique to Prove Borel's Strong Law of Large Numbers Wen, L. Am Math Month 1991]</ref> |
|||
: <math> \frac{N_n(E)}{n}\to p\text{ as }n\to\infty.</math> |
|||
This theorem makes rigorous the intuitive notion of probability as the long-run relative frequency of an event's occurrence. It is a special case of any of several more general laws of large numbers in probability theory. |
|||
'''[[Chebyshev's inequality]]'''. Let ''X'' be a [[random variable]] with finite [[expected value]] ''μ'' and finite non-zero [[variance]] ''σ''<sup>2</sup>. Then for any [[real number]] {{nowrap|''k'' > 0}}, |
|||
: <math> |
|||
\Pr(|X-\mu|\geq k\sigma) \leq \frac{1}{k^2}. |
|||
</math> |
|||
==Proof of the weak law== |
|||
Given ''X''<sub>1</sub>, ''X''<sub>2</sub>, ... an infinite sequence of [[i.i.d.]] random variables with finite expected value ''E''(''X''<sub>1</sub>) = ''E''(''X''<sub>2</sub>) = ... = µ < ∞, we are interested in the convergence of the sample average |
|||
:<math>\overline{X}_n=\tfrac1n(X_1+\cdots+X_n). </math> |
|||
The weak law of large numbers states: |
|||
{{NumBlk||'''Theorem:''' <math>\begin{matrix}{}\\ |
|||
\overline{X}_n\ \xrightarrow{P}\ \mu \qquad\textrm{when}\ n \to \infty. |
|||
\\{}\end{matrix}</math>|{{EquationNote|law. 2}}}} |
|||
===Proof using Chebyshev's inequality assuming finite variance=== |
|||
This proof uses the assumption of finite [[variance]] <math> \operatorname{Var} (X_i)=\sigma^2 </math> (for all <math>i</math>). The independence of the random variables implies no correlation between them, and we have that |
|||
:<math> |
|||
\operatorname{Var}(\overline{X}_n) = \operatorname{Var}(\tfrac1n(X_1+\cdots+X_n)) = \frac{1}{n^2} \operatorname{Var}(X_1+\cdots+X_n) = \frac{n\sigma^2}{n^2} = \frac{\sigma^2}{n}. |
|||
</math> |
|||
The common mean μ of the sequence is the mean of the sample average: |
|||
:<math> |
|||
E(\overline{X}_n) = \mu. |
|||
</math> |
|||
Using [[Chebyshev's inequality]] on <math>\overline{X}_n </math> results in |
|||
:<math> |
|||
\operatorname{P}( \left| \overline{X}_n-\mu \right| \geq \varepsilon) \leq \frac{\sigma^2}{n\varepsilon^2}. |
|||
</math> |
|||
This may be used to obtain the following: |
|||
:<math> |
|||
\operatorname{P}( \left| \overline{X}_n-\mu \right| < \varepsilon) = 1 - \operatorname{P}( \left| \overline{X}_n-\mu \right| \geqslant \varepsilon) \geqslant 1 - \frac{\sigma^2}{n \varepsilon^2 }. |
|||
</math> |
|||
As ''n'' approaches infinity, the expression approaches 1. And by definition of [[convergence in probability]], we have obtained |
|||
{{NumBlk|:|<math>\begin{matrix}{}\\ |
|||
\overline{X}_n\ \xrightarrow{P}\ \mu \qquad\textrm{when}\ n \to \infty. |
|||
\\{}\end{matrix}</math>|{{EquationNote|law. 2}}}} |
|||
===Proof using convergence of characteristic functions=== |
|||
By [[Taylor's theorem]] for [[complex function]]s, the [[Characteristic function (probability theory)|characteristic function]] of any random variable, ''X'', with finite mean μ, can be written as |
|||
:<math>\varphi_X(t) = 1 + it\mu + o(t), \quad t \rightarrow 0.</math> |
|||
All ''X''<sub>1</sub>, ''X''<sub>2</sub>, ... have the same characteristic function, so we will simply denote this ''φ''<sub>''X''</sub>. |
|||
Among the basic properties of characteristic functions there are |
|||
:<math>\varphi_{\frac 1 n X}(t)= \varphi_X(\tfrac t n) \quad \text{and} \quad |
|||
\varphi_{X+Y}(t)=\varphi_X(t) \varphi_Y(t) \quad </math> if ''X'' and ''Y'' are independent. |
|||
These rules can be used to calculate the characteristic function of <math>\scriptstyle\overline{X}_n</math> in terms of ''φ''<sub>''X''</sub>: |
|||
:<math>\varphi_{\overline{X}_n}(t)= \left[\varphi_X\left({t \over n}\right)\right]^n = \left[1 + i\mu{t \over n} + o\left({t \over n}\right)\right]^n \, \rightarrow \, e^{it\mu}, \quad \text{as} \quad n \rightarrow \infty.</math> |
|||
The limit ''e''<sup>''it''μ</sup> is the characteristic function of the constant random variable μ, and hence by the [[Lévy continuity theorem]], <math> \scriptstyle\overline{X}_n</math> [[Convergence in distribution|converges in distribution]] to μ: |
|||
:<math>\overline{X}_n \, \xrightarrow{\mathcal D} \, \mu \qquad\text{for}\qquad n \to \infty.</math> |
|||
μ is a constant, which implies that convergence in distribution to μ and convergence in probability to μ are equivalent (see [[Convergence of random variables]].) Therefore, |
|||
{{NumBlk|:|<math>\begin{matrix}{}\\ |
|||
\overline{X}_n\ \xrightarrow{P}\ \mu \qquad\textrm{when}\ n \to \infty. |
|||
\\{}\end{matrix}</math>|{{EquationNote|law. 2}}}} |
|||
This shows that the sample mean converges in probability to the derivative of the characteristic function at the origin, as long as the latter exists. |
|||
== Vegeu també == |
== Vegeu també == |
||
* [[Funció característica (teoria de la probabilitat)#Llei feble dels grans nombres|Llei feble dels grans nombres]] |
* [[Funció característica (teoria de la probabilitat)#Llei feble dels grans nombres|Llei feble dels grans nombres]] |
||
==Notes== |
|||
{{Reflist|2}} |
|||
==References== |
|||
{{refbegin}} |
|||
* {{cite book |author1=Grimmett, G. R. |author2=Stirzaker, D. R. | title=Probability and Random Processes, 2nd Edition | publisher=Clarendon Press, Oxford | year=1992 | isbn=0-19-853665-8}} |
|||
* {{cite book | author=Richard Durrett | title=Probability: Theory and Examples, 2nd Edition | publisher=Duxbury Press | year=1995}} |
|||
* {{cite book | author=Martin Jacobsen | publisher= HCØ-tryk, Copenhagen | year=1992|title=Videregående Sandsynlighedsregning (Advanced Probability Theory) 3rd Edition| isbn=87-91180-71-6}} |
|||
* {{cite book |
|||
| last = Loève | first = Michel |
|||
| title = Probability theory 1 |
|||
| year = 1977 |
|||
| edition = 4th |
|||
| publisher = Springer Verlag |
|||
| ref = CITEREFLo.C3.A8ve1977 |
|||
}} |
|||
* {{cite book |
|||
| last1 = Newey | first1 = Whitney K. |
|||
| last2 = McFadden | first2 = Daniel | authorlink2 = Daniel McFadden |
|||
| title = Large sample estimation and hypothesis testing |
|||
| series = Handbook of econometrics, vol. IV, Ch. 36 |
|||
| year = 1994 |
|||
| publisher = Elsevier Science |
|||
| pages = 2111–2245 |
|||
| ref = CITEREFNeweyMcFadden1994 |
|||
}} |
|||
* {{cite book |
|||
| last = Ross | first = Sheldon |
|||
| title = A first course in probability |
|||
| year = 2009 |
|||
| edition = 8th |
|||
| publisher = Prentice Hall press |
|||
| isbn = 978-0-13-603313-4 |
|||
}} |
|||
* {{cite book |
|||
| last1 = Sen | first1 = P. K |
|||
| last2 = Singer | first2 = J. M. |
|||
| year = 1993 |
|||
| title = Large sample methods in statistics |
|||
| publisher = Chapman & Hall, Inc |
|||
| ref = CITEREFSenSinger1993 |
|||
}} |
|||
* {{citation|last=Seneta|first=Eugene|title=A Tricentenary history of the Law of Large Numbers| journal=Bernoulli| volume=19| issue=4| pages=1088–1121| date=2013|doi=10.3150/12-BEJSP12|arxiv=1309.6488}} |
|||
{{refend}} |
|||
==External links== |
|||
* {{springer|title=Law of large numbers|id=p/l057720}} |
|||
* {{MathWorld|urlname=WeakLawofLargeNumbers|title=Weak Law of Large Numbers}} |
|||
* {{MathWorld|urlname=StrongLawofLargeNumbers|title=Strong Law of Large Numbers}} |
|||
* [https://web.archive.org/web/20081110071309/http://animation.yihui.name/prob:law_of_large_numbers Animations for the Law of Large Numbers] by Yihui Xie using the [[R (programming language)|R]] package [https://cran.r-project.org/package=animation animation] |
|||
* [http://www.businessinsider.com/law-of-large-numbers-tim-cook-2015-2 Apple CEO Tim Cook said something that would make statisticians cringe]. "We don't believe in such laws as laws of large numbers. This is sort of, uh, old dogma, I think, that was cooked up by somebody [..]" said Tim Cook and while: "However, the law of large numbers has nothing to do with large companies, large revenues, or large growth rates. The law of large numbers is a fundamental concept in probability theory and statistics, tying together theoretical probabilities that we can calculate to the actual outcomes of experiments that we empirically perform.'' explained [[Business Insider]]'' |
|||
{{esborrany de matemàtiques}} |
{{esborrany de matemàtiques}} |
Revisió del 03:56, 19 nov 2019
![]() |
Aquest article o secció no cita les fonts o necessita més referències per a la seva verificabilitat. |
![]() |
Aquest article o secció s'està traduint a partir de: «Law of large numbers» (anglès) en la versió del 18/11/2019, amb llicència CC-BY-SA Hi pot haver llacunes de contingut, errors sintàctics o escrits sense traduir. |

En teoria de la probabilitat, la llei dels grans nombres és un teorema segons el qual quan el nombre d'observacions d'un fenomen aleatori és molt gran, la freqüència d'un esdeveniment associat amb aquest s'aproxima progressivament a un valor determinat. Aquest valor s'anomena probabilitat de l'esdeveniment.
Gràcies a aquesta llei podem, experimentalment:
- Comprovar si són vàlides o no les probabilitats assignades a priori als esdeveniments dels instruments aleatoris suposadament regulars.
- Obtenir de manera aproximada les probabilitats d'esdeveniments d'experiències aleatòries irregulars.
Aquesta llei és important perquè garanteix relacions estables entre les mitjanes de diversos esdeveniments aleatoris. Per exemple, mentre que un casino pot perdre diners en una simple tirada de la ruleta, els seus guanys tendiran a un percentatge predictible amb un nombre gran de tirades. Qualsevol tongada sort del jugador serà, eventualment, superada pels paràmetres del joc. Cal recordar que la llei, però, tan sols s'aplica quan es considera un nombre elevat d'observacions, tal com el nom indica. El principi no es pot aplicar per un nombre petit d'observacions ni es pot esperar que una tongada d'un valor concret sigui immediatament "equilibrada" amb l'obtenció d'altres valors (consulta la fal·làcia del jugador).
Exemples
Per exemple, una única tirada d'un dau equilibrat de sis cares té sis possibles resultats (1, 2, 3, 4, 5 o 6), tots amb la mateixa probabilitat. Per tant, el valor esperat de la mitjana de tirades és de:
Segons la llei dels grans nombres, si es tiren un nombre elevat de daus de 6 cares, la mitjana dels seus valors serà pròxima a 3.5 i la seva precisió augmentarà amb la tirada de més daus.
D'aquesta llei, se'n pot deduir que la probabilitat empírica d'un succés o esdeveniment en una sèrie d'assajos de Bernoulli convergiran a la probabilitat teòrica. Per una variable aleatòria de Bernoulli, el valor esperat després d'un nombre d'assajos prou elevat coincideix amb la probabilitat teòrica de l'esdeveniment i la mitjana de les n variables (assumint que són aleatòries, independents i idènticament distribuïdes (i.i.d.)) çes precisament la freqüència relativa.
Per exemple, la tirada d'una moneda equilibrada (on la probabilitat d'obtenir cada cara és igual), és un assaig de Bernoulli. Quan es tira per primer cop, la probabilitat de cara és 1/2. Per tant, segons la llei dels grans nombres, la proporció de cares en un nombre "gran" de tirades "hauria de ser" aproximadament 1/2. En concret, la proporció de cares després de n tirades convergirà de forma quasisegura cap a 1/2 a mesura que n s'apropa a infinit.
Malgrat que la proporció de cara (i creu) s'apropa a 1/2, a mesura que creix el nombre de tirades, pràcticament segur que la diferència absoluta entre el nombre de cares i creus també creixerà. D'altra banda, també pràcticament segur que el ràtio de la diferència absoluta respecte el nombre de tirades s'aproximarà a zero. De forma inuïtiva, la diferència absoluta esperada creix, però a un ritme menor que el nombre de tirades.
History
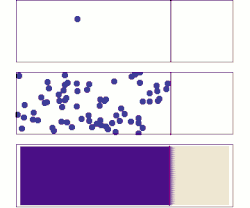
Top: With a single molecule, the motion appears to be quite random.
Middle: With more molecules, there is clearly a trend where the solute fills the container more and more uniformly, but there are also random fluctuations.
Bottom: With an enormous number of solute molecules (too many to see), the randomness is essentially gone: The solute appears to move smoothly and systematically from high-concentration areas to low-concentration areas. In realistic situations, chemists can describe diffusion as a deterministic macroscopic phenomenon (see Fick's laws), despite its underlying random nature.
El matemàtic italià Gerolamo Cardano (1501–1576) va constatar, sense demostrar, que la precisió de les mesures estadístiques empíriques tendien a millorar amb el nombre d'assajos[1] i es va formalitzar com a llei dels grans nombres. Una forma especial d'aquesta llei, per les variables aleatòries binàries) va ser demostrar primerament per Jacob Bernoulli.[2] Li va costar més de 20 anys desenvolupar una prova matemàtica suficientment rigorosa, que va ser publicada en la seva obra Ars Conjectandi (L'art de la conjentura) el 1713. El va anomenar el seu "Teorema daurat", però va ser conegut generalment amb el nom del "Teorema de Bernoulli", que no s'ha de confondre amb el Principi de Bernoulli, referit al seu cosí Daniel Bernoulli. El 1837, S.D. Poisson el va desenvolupar més sota el nom "la loi des grands nombres" ("La llei dels grans nombres").[3][4] D'aleshores encà, es coneix pels dos noms, tot i que la "Llei dels grans nombres" és més freqüent.
Després que Benroulli i Poisson publiquessin els seus esforços, altres matemàtics van contribuir a refinar la llei, com Chebyshev,[5] Markov, Borel, Cantelli and Kolmogorov i Khinchin. Markov va demostrar que la llei es podia aplicar a variables aleatòries que no tinguessin una variància finita sota alguna altra hipòtesi més feble, i Khinchin va provar, el 1929, que si la sèrie consistia en variables aleatòries independents i idènticament distribuides, era suficient que el valor esperat existis per tal que la llei feble dels grans nombres fos veritat.[6] Com a conseqüència d'aquests nous estudis, van sorgir dos formes prominents de la Llei dels grans nombres. Una anomenada la llei "feble" i l'altra la llei "forta", en referència a dos formes diferents de la convergència de la mitjana cumulativa de mostres cap al valor infinit. Tal com s'explica més endavant, la forma forta implica la feble.[7]
Formes
Existeixen dues versions diferents de la llei dels grans nombres: la llei forta dels grans nombres i la llei feble dels grans nombres. Per una seqüència infinita de variables aleatòries Lebesgue integrables X1, X2, ... amb valor esperat E(X1) = E(X2) = ...= µ, ambdues versions de la llei afirmen que, amb certesa virtual, la mitjana mostral, definida com a
convergeix al valor esperat
-
(
)
La integrabilitat de Lebesgue per Xj significa que el valor esperat E(Xj) existeix seguint la Integral de Lebesgue i és finit. No implica que la mesura de probabilitat associada sigui continua absolutament respecte la mesura de Lebesgue.
La hipòtesi de variància finita Var(X1) = Var(X2) = ... = σ2 < ∞ no és necessària. Una variància gran o infinita farà que la convergència sigui més lenta, però la llei dels grans nombres es manté de tota manera. Aquesta hipòtesi, però, sovint s'usa per tal de fer la demostració més fàcil i més curta.
La independència mútua de les variables aleatòries pot ser substituïda per la independència parell a en ambdues versoins de la llei.[8]
La diferència entre la versió de la llei feble i la forta rau en la manera en què la convergència és definida.
Llei feble

The weak law of large numbers (also called Khinchin's law) states that the sample average converges in probability towards the expected value[9]
-
(
)
That is, for any positive number ε,
Interpreting this result, the weak law states that for any nonzero margin specified, no matter how small, with a sufficiently large sample there will be a very high probability that the average of the observations will be close to the expected value; that is, within the margin.
As mentioned earlier, the weak law applies in the case of i.i.d. random variables, but it also applies in some other cases. For example, the variance may be different for each random variable in the series, keeping the expected value constant. If the variances are bounded, then the law applies, as shown by Chebyshev as early as 1867. (If the expected values change during the series, then we can simply apply the law to the average deviation from the respective expected values. The law then states that this converges in probability to zero.) In fact, Chebyshev's proof works so long as the variance of the average of the first n values goes to zero as n goes to infinity.[6] As an example, assume that each random variable in the series follows a Gaussian distribution with mean zero, but with variance equal to , which is not bounded. At each stage, the average will be normally distributed (as the average of a set of normally distributed variables). The variance of the sum is equal to the sum of the variances, which is asymptotic to . The variance of the average is therefore asymptotic to and goes to zero.
An example where the law of large numbers does not apply is the Cauchy distribution. Another example is where the random numbers equal the tangent of an angle uniformly distributed between −90° and +90°. The median is zero, but the expected value does not exist, and indeed the average of n such variables has the same distribution as one such variable. It does not converge in probability towards zero (or any other value) as n goes to infinity.
There are also examples of the weak law applying even though the expected value does not exist. See #Differences between the weak law and the strong law.
Strong law
The strong law of large numbers states that the sample average converges almost surely to the expected value[10]
-
(
)
That is,
What this means is that the probability that, as the number of trials n goes to infinity, the average of the observations converges to the expected value, is equal to one.
The proof is more complex than that of the weak law.[11] This law justifies the intuitive interpretation of the expected value (for Lebesgue integration only) of a random variable when sampled repeatedly as the "long-term average".
Almost sure convergence is also called strong convergence of random variables. This version is called the strong law because random variables which converge strongly (almost surely) are guaranteed to converge weakly (in probability). The strong law implies the weak law but not vice versa, when the strong law conditions hold the variable converges both strongly (almost surely) and weakly (in probability). However the weak law is known to hold in certain conditions where the strong law does not hold and then the convergence is only weak (in probability).[Cal aclariment]
The strong law of large numbers can itself be seen as a special case of the pointwise ergodic theorem.
The strong law applies to independent identically distributed random variables having an expected value (like the weak law). This was proved by Kolmogorov in 1930. It can also apply in other cases. Kolmogorov also showed, in 1933, that if the variables are independent and identically distributed, then for the average to converge almost surely on something (this can be considered another statement of the strong law), it is necessary that they have an expected value (and then of course the average will converge almost surely on that).[12]
If the summands are independent but not identically distributed, then
provided that each Xk has a finite second moment and
This statement is known as Kolmogorov's strong law, see e.g. Sen & Singer (1993, Theorem 2.3.10).
An example of a series where the weak law applies but not the strong law is when Xk is plus or minus (starting at sufficiently large k so that the denominator is positive) with probability 1/2 for each.[12] The variance of Xk is then Kolmogorov's strong law does not apply because the partial sum in his criterion up to k=n is asymptotic to and this is unbounded.
If we replace the random variables with Gaussian variables having the same variances, namely then the average at any point will also be normally distributed. The width of the distribution of the average will tend toward zero (standard deviation asymptotic to ), but for a given ε, there is probability which does not go to zero with n that the average sometime after the nth trial will come back up to ε. Since this probability does not go to zero [Cal aclariment], it must have a positive lower bound p(ε), which means there is a probability of at least p(ε) that the average will attain ε after n trials. It will happen with probability p(ε)/2 before some m which depends on n. But even after m, there is still a probability of at least p(ε) that it will happen. (This seems to indicate that p(ε)=1 and the average will attain ε an infinite number of times.)
Differences between the weak law and the strong law
The weak law states that for a specified large n, the average is likely to be near μ. Thus, it leaves open the possibility that happens an infinite number of times, although at infrequent intervals. (Not necessarily for all n).
The strong law shows that this almost surely will not occur. In particular, it implies that with probability 1, we have that for any ε > 0 the inequality holds for all large enough n.[13]
The strong law does not hold in the following cases, but the weak law does.[14][15][16]
1. Let X be an exponentially distributed random variable with parameter 1. The random variable has no expected value according to Lebesgue integration, but using conditional convergence and interpreting the integral as a Dirichlet integral, which is an improper Riemann integral, we can say:
2. Let x be geometric distribution with probability 0.5. The random variable does not have an expected value in the conventional sense because the infinite series is not absolutely convergent, but using conditional convergence, we can say:
3. If the cumulative distribution function of a random variable is
Uniform law of large numbers
Suppose f(x,θ) is some function defined for θ ∈ Θ, and continuous in θ. Then for any fixed θ, the sequence {f(X1,θ), f(X2,θ), ...} will be a sequence of independent and identically distributed random variables, such that the sample mean of this sequence converges in probability to E[f(X,θ)]. This is the pointwise (in θ) convergence.
The uniform law of large numbers states the conditions under which the convergence happens uniformly in θ. If[19][20]
- Θ is compact,
- f(x,θ) is continuous at each θ ∈ Θ for almost all xs, and measurable function of x at each θ.
- there exists a dominating function d(x) such that E[d(X)] < ∞, and
Then E[f(X,θ)] is continuous in θ, and
This result is useful to derive consistency of a large class of estimators (see Extremum estimator).
Borel's law of large numbers
Borel's law of large numbers, named after Émile Borel, states that if an experiment is repeated a large number of times, independently under identical conditions, then the proportion of times that any specified event occurs approximately equals the probability of the event's occurrence on any particular trial; the larger the number of repetitions, the better the approximation tends to be. More precisely, if E denotes the event in question, p its probability of occurrence, and Nn(E) the number of times E occurs in the first n trials, then with probability one,[21]
This theorem makes rigorous the intuitive notion of probability as the long-run relative frequency of an event's occurrence. It is a special case of any of several more general laws of large numbers in probability theory.
Chebyshev's inequality. Let X be a random variable with finite expected value μ and finite non-zero variance σ2. Then for any real number k > 0,
Proof of the weak law
Given X1, X2, ... an infinite sequence of i.i.d. random variables with finite expected value E(X1) = E(X2) = ... = µ < ∞, we are interested in the convergence of the sample average
The weak law of large numbers states:
Theorem: |
|
(law. 2) |
Proof using Chebyshev's inequality assuming finite variance
This proof uses the assumption of finite variance (for all ). The independence of the random variables implies no correlation between them, and we have that
The common mean μ of the sequence is the mean of the sample average:
Using Chebyshev's inequality on results in
This may be used to obtain the following:
As n approaches infinity, the expression approaches 1. And by definition of convergence in probability, we have obtained
-
(law. 2)
Proof using convergence of characteristic functions
By Taylor's theorem for complex functions, the characteristic function of any random variable, X, with finite mean μ, can be written as
All X1, X2, ... have the same characteristic function, so we will simply denote this φX.
Among the basic properties of characteristic functions there are
- if X and Y are independent.
These rules can be used to calculate the characteristic function of in terms of φX:
The limit eitμ is the characteristic function of the constant random variable μ, and hence by the Lévy continuity theorem, converges in distribution to μ:
μ is a constant, which implies that convergence in distribution to μ and convergence in probability to μ are equivalent (see Convergence of random variables.) Therefore,
-
(law. 2)
This shows that the sample mean converges in probability to the derivative of the characteristic function at the origin, as long as the latter exists.
Vegeu també
Notes
- ↑ Mlodinow, L. The Drunkard's Walk. New York: Random House, 2008. p. 50.
- ↑ Jakob Bernoulli, Ars Conjectandi: Usum & Applicationem Praecedentis Doctrinae in Civilibus, Moralibus & Oeconomicis, 1713, Chapter 4, (Translated into English by Oscar Sheynin)
- ↑ Poisson names the "law of large numbers" (la loi des grands nombres) in: S.D. Poisson, Probabilité des jugements en matière criminelle et en matière civile, précédées des règles générales du calcul des probabilitiés (Paris, France: Bachelier, 1837), p. 7. He attempts a two-part proof of the law on pp. 139–143 and pp. 277 ff.
- ↑ Hacking, Ian. (1983) "19th-century Cracks in the Concept of Determinism", Journal of the History of Ideas, 44 (3), 455-475 Plantilla:Jstor
- ↑ Tchebichef, P. «Démonstration élémentaire d'une proposition générale de la théorie des probabilités». Journal für die reine und angewandte Mathematik, vol. 1846, 33, 1846, pàg. 259–267. DOI: 10.1515/crll.1846.33.259.
- ↑ 6,0 6,1 «Law of large numbers». Encyclopedia of Mathematics.
- ↑ Seneta, 2013.
- ↑ Etemadi, N.Z. «An elementary proof of the strong law of large numbers». Wahrscheinlichkeitstheorie Verw Gebiete, vol. 55, 1, 1981, pàg. 119–122. DOI: 10.1007/BF01013465.
- ↑ Loève 1977, Chapter 1.4, p. 14
- ↑ Loève 1977, Chapter 17.3, p. 251
- ↑ «The strong law of large numbers – What's new». Terrytao.wordpress.com. [Consulta: 9 juny 2012].
- ↑ 12,0 12,1 «Strong law of large numbers». Encyclopedia of Mathematics.
- ↑ Ross (2009)
- ↑ Lehmann, Erich L; Romano, Joseph P. Weak law converges to constant, 2006-03-30. ISBN 9780387276052.
- ↑ «A NOTE ON THE WEAK LAW OF LARGE NUMBERS FOR EXCHANGEABLE RANDOM VARIABLES». Dguvl Hun Hong and Sung Ho Lee.
- ↑ «weak law of large numbers: proof using characteristic functions vs proof using truncation VARIABLES».
- ↑ Mukherjee, Sayan. «Law of large numbers». Arxivat de l'original el 2013-03-09. [Consulta: 28 juny 2014].
- ↑ J. Geyer, Charles. «Law of large numbers».
- ↑ Newey & McFadden 1994, Lemma 2.4
- ↑ Jennrich, Robert I. «Asymptotic Properties of Non-Linear Least Squares Estimators». The Annals of Mathematical Statistics, vol. 40, 2, 1969, pàg. 633–643. DOI: 10.1214/aoms/1177697731.
- ↑ An Analytic Technique to Prove Borel's Strong Law of Large Numbers Wen, L. Am Math Month 1991
References
- Grimmett, G. R.. Probability and Random Processes, 2nd Edition. Clarendon Press, Oxford, 1992. ISBN 0-19-853665-8.
- Richard Durrett. Probability: Theory and Examples, 2nd Edition. Duxbury Press, 1995.
- Martin Jacobsen. Videregående Sandsynlighedsregning (Advanced Probability Theory) 3rd Edition. HCØ-tryk, Copenhagen, 1992. ISBN 87-91180-71-6.
- Loève, Michel. Probability theory 1. 4th. Springer Verlag, 1977.
- Newey, Whitney K.; McFadden, Daniel. Large sample estimation and hypothesis testing. Elsevier Science, 1994, p. 2111–2245 (Handbook of econometrics, vol. IV, Ch. 36).
- Ross, Sheldon. A first course in probability. 8th. Prentice Hall press, 2009. ISBN 978-0-13-603313-4.
- Sen, P. K; Singer, J. M.. Large sample methods in statistics. Chapman & Hall, Inc, 1993.
- Seneta, Eugene (2013), "A Tricentenary history of the Law of Large Numbers", Bernoulli 19 (4): 1088–1121, DOI 10.3150/12-BEJSP12
External links
- Michiel Hazewinkel (ed.). Law of large numbers. Encyclopedia of Mathematics (en anglès). Springer, 2001. ISBN 978-1-55608-010-4.
- Weisstein, Eric W., «Weak Law of Large Numbers» a MathWorld (en anglès).
- Weisstein, Eric W., «Strong Law of Large Numbers» a MathWorld (en anglès).
- Animations for the Law of Large Numbers by Yihui Xie using the R package animation
- Apple CEO Tim Cook said something that would make statisticians cringe. "We don't believe in such laws as laws of large numbers. This is sort of, uh, old dogma, I think, that was cooked up by somebody [..]" said Tim Cook and while: "However, the law of large numbers has nothing to do with large companies, large revenues, or large growth rates. The law of large numbers is a fundamental concept in probability theory and statistics, tying together theoretical probabilities that we can calculate to the actual outcomes of experiments that we empirically perform. explained Business Insider